Betrayed by Captions: Joint Caption Grounding and Generation for Open Vocabulary Instance Segmentation
arXiv, 2023
Paper
Abstract
In this work, we focus on open vocabulary instance segmentation to expand a segmentation model to classify and segment instance-level novel categories. Previous approaches have relied on massive caption datasets and complex pipelines to establish one-to-one mappings between image regions and words in captions. However, such methods build noisy supervision by matching non-visible words to image regions, such as adjectives and verbs. Meanwhile, context words are also important for inferring the existence of novel objects as they show high inter-correlations with novel categories. To overcome these limitations, we devise a joint Caption Grounding and Generation (CGG) framework, which incorporates a novel grounding loss that only focuses on matching object nouns to improve learning efficiency. We also introduce a caption generation head that enables additional supervision and contextual modeling as a complementation to the grounding loss. Our analysis and results demonstrate that grounding and generation components complement each other, significantly enhancing the segmentation performance for novel classes. Experiments on the COCO dataset with two settings: Open Vocabulary Instance Segmentation (OVIS) and Open Set Panoptic Segmentation (OSPS) demonstrate the superiority of the CGG. Specifically, CGG achieves a substantial improvement of 6.8% mAP for novel classes without extra data on the OVIS task and 15% PQ improvements for novel classes on the OSPS benchmark.
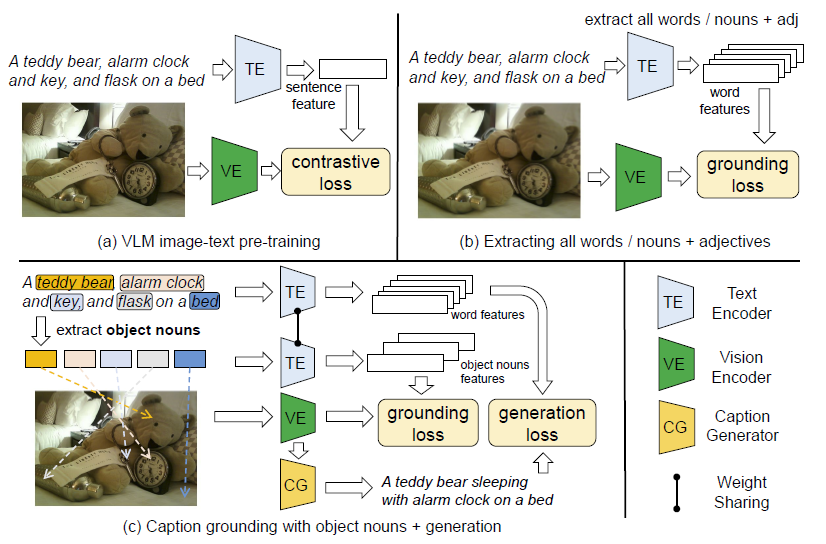
(a), VLMs learn image-level visual-linguistic alignment using caption data. (b), Previous open vocabulary detection/segmentation methods extract all words or nouns + adjectives for caption grounding. (c), The proposed CGG extracts object nouns for a finer alignment between objects in the caption and visible entities in the image and then combines a caption generation loss to fully utilize the contextual knowledge in the caption.
Motivation
Object Nouns are more important!
We find the previous works (both (a) and (b)) suffer from the problem that invisible nouns (room in the example) are learned to be aligned by the multi-modal embeddings, while using object nouns avoids the question. We adopt top-10 object queries according to their object scores. This motivates us to reformulate the ground loss by only focusing on object nouns.

A comparison analysis of caption grounding using different types of words. The color maps are normalized similarities between multi-modal embeddings and word features extracted by the language encoder.
Motivation
The caption data encode the co-occurrence relation of novel class!
We observe certain pairs of words co-occurrence often, while others do not. We calculate the co-occurrence matrix between novel classes and frequent words in the caption. Different classes have various distributions on co-occurrence words.
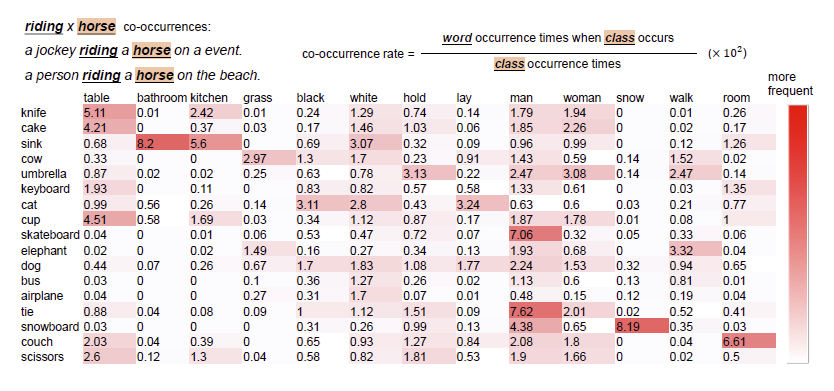
Framework
Caption Grounding and Generation
Following CLIP, we set the pre-trained text embeddings as the weights of the linear classifier. Then we add two losses: the caption grounding loss and the caption generation loss. A caption generator is appended at the end of the output queries, producing the image caption. During training, we adopt a pre-trained sentence encoder and word encoder to encode both captions and object nouns extracted from captions into sentence and word features.
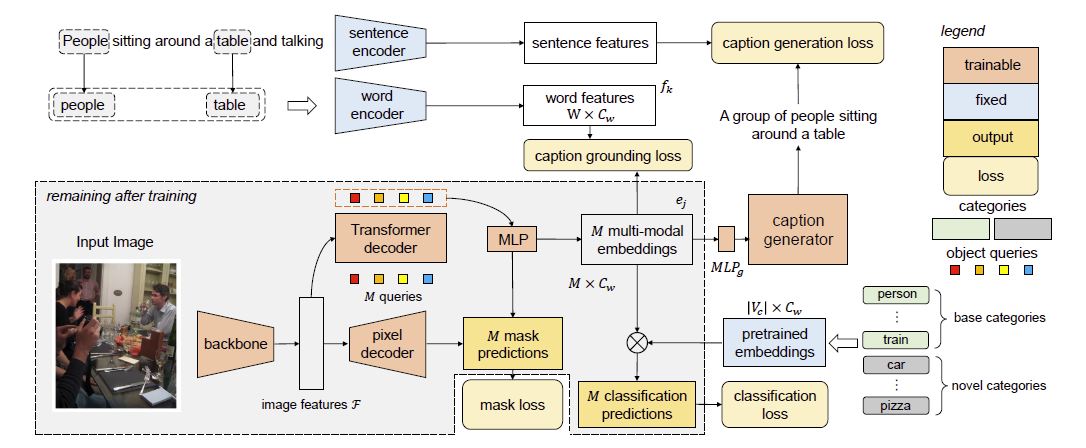
Experimental
Results
Method | Constrained-Base | Constrained-Novel | Generalized-Base | Generalized-Novel | Generalized-All |
---|---|---|---|---|---|
OVR | 42.0 | 20.9 | 41.6 | 17.1 | 35.2 |
SB | 41.6 | 20.8 | 41.0 | 16.0 | 34.5 |
BA-RPN | 41.8 | 20.1 | 41.3 | 15.4 | 34.5 |
XPM | 42.4 | 24.0 | 41.5 | 21.6 | 36.3 |
CGG (ours) | 46.8 | 29.5 | 46.0 | 28.4 | 41.4 |
Method | Unknown Ratio | Known Classes | Unknown Classes | ||||
---|---|---|---|---|---|---|---|
PQTh | SQTh | PQSt | SQSt | PQTh | SQTh | ||
EOPSN | 5% | 44.8 | 80.5 | 28.3 | 73.1 | 23.1 | 74.7 |
Dual | 5% | 45.1 | 80.9 | 28.1 | 73.1 | 30.2 | 80.0 |
CGG (ours) | 5% | 50.2 | 83.1 | 34.3 | 81.5 | 45.0 | 85.2 |
EOPSN | 10% | 44.5 | 80.6 | 28.1 | 73.1 | 30.2 | 80.0 |
Dual | 10% | 45.0 | 80.7 | 27.8 | 72.2 | 24.5 | 79.9 |
CGG (ours) | 10% | 49.2 | 82.8 | 34.6 | 81.2 | 41.6 | 82.6 |
EOPSN | 15% | 45.0 | 80.3 | 28.2 | 71.2 | 11.3 | 73.8 |
Dual | 15% | 45.0 | 80.6 | 27.6 | 70.1 | 21.4 | 79.1 |
CGG (ours) | 15% | 48.4 | 82.3 | 34.4 | 81.1 | 36.5 | 78.0 |
VISUALIZATION
Examples
We show the novel classes segmentation on both open vocabulary instance segmentation and open set panoptic segmetnation. We also generate captions for each image-prediction pair and highlight the novel categories in the captions, if any.
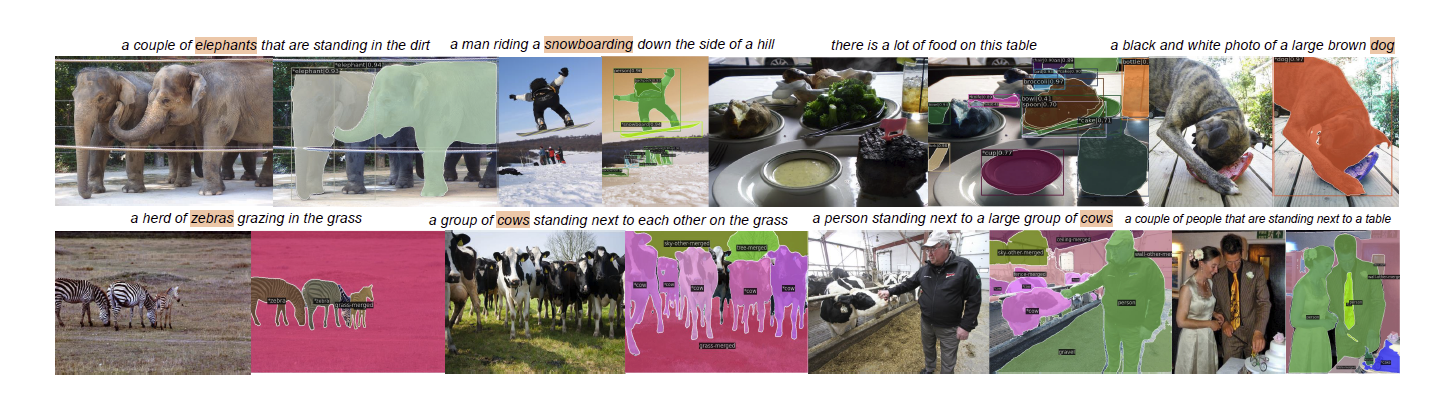
Visualization results on open vocabulary instance segmentation (Top) and open set panoptic segmentation (Bottom). The categories with “*” are novel
Paper
Citation
@article{wu2023betrayed,
title={Betrayed by Captions: Joint Caption Grounding and Generation for Open Vocabulary Instance Segmentation},
author={Wu, Jianzong and Li, Xiangtai and Ding, Henghui and Li, Xia and Cheng, Guangliang and Tong, Yunhai and Loy, Chen Change},
journal={arXiv preprint arXiv:2301.00805},
year={2023}
}
Related
Projects
-
Video K-Net: A Simple, Strong, and Unified Baseline For End-to-End Dense Video Segmentation
X. Li, W. Zhang, J. Pang, K. Chen, G. Cheng, Y. Tong, C. C. Loy
in Proceedings of IEEE Conference on Computer Vision and Pattern Recognition, 2022 (CVPR, Oral)
[PDF] [arXiv] [Supplementary Material] [Project Page] -
Tube-Link: A Flexible Cross Tube Baseline for Universal Video Segmentation
X. Li, H. Yuan, W. Zhang, G. Cheng, J. Pang, C. C. Loy
Technical report, arXiv:2303.12782, 2023
[arXiv] [Project Page]
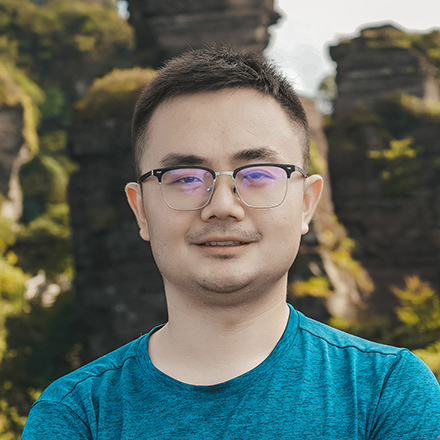