Pastiche Master: Exemplar-Based High-Resolution Portrait Style Transfer
CVPR 2022
Paper
Abstract
Recent studies on StyleGAN show high performance on artistic portrait generation by transfer learning with limited data. In this paper, we explore more challenging exemplar-based high-resolution portrait style transfer by introducing a novel DualStyleGAN with flexible control of dual styles of the original face domain and the extended artistic portrait domain. Different from StyleGAN, DualStyleGAN provides a natural way of style transfer by characterizing the content and style of a portrait with an intrinsic style path and a new extrinsic style path, respectively. The delicately designed extrinsic style path enables our model to modulate both the color and complex structural styles hierarchically to precisely pastiche the style example. Furthermore, a novel progressive fine-tuning scheme is introduced to smoothly transform the generative space of the model to the target domain, even with the above modifications on the network architecture. Experiments demonstrate the superiority of DualStyleGAN over state-of-the-art methods in high-quality portrait style transfer and flexible style control.
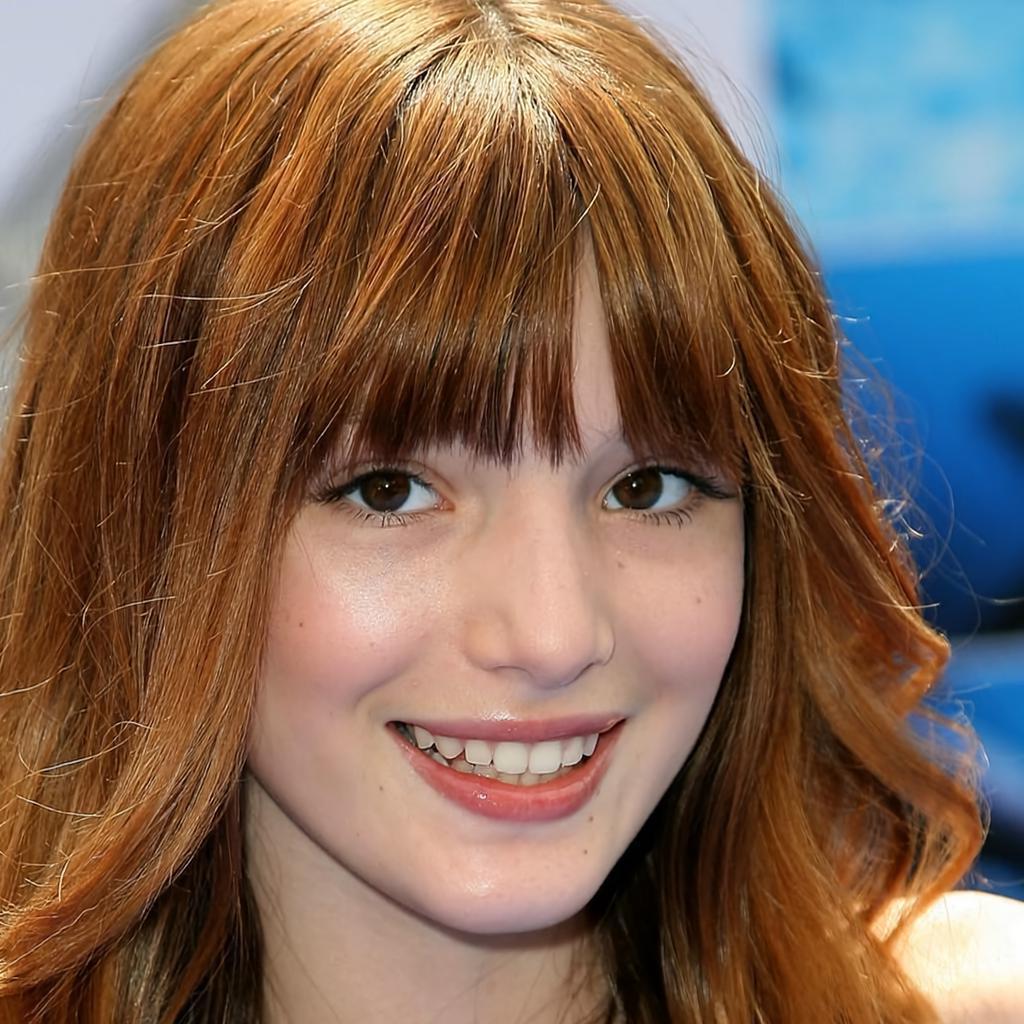
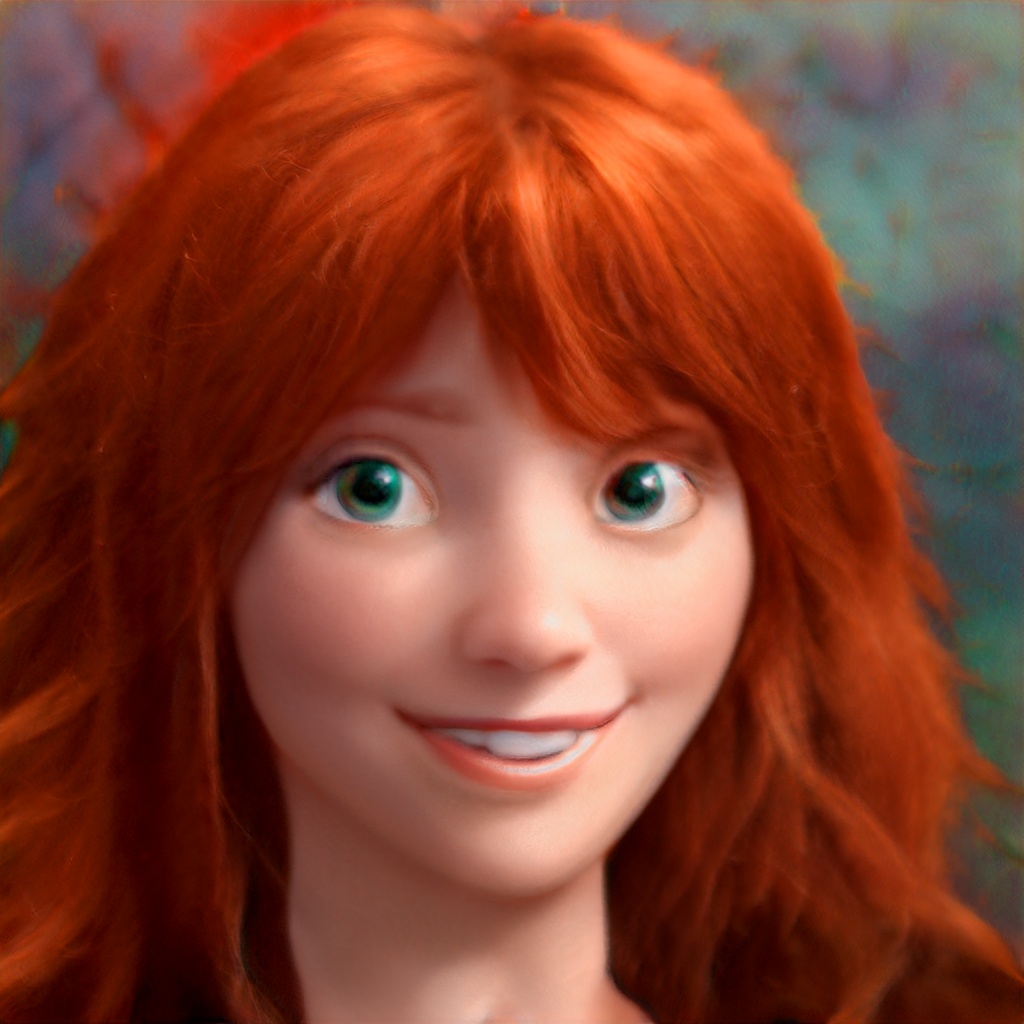
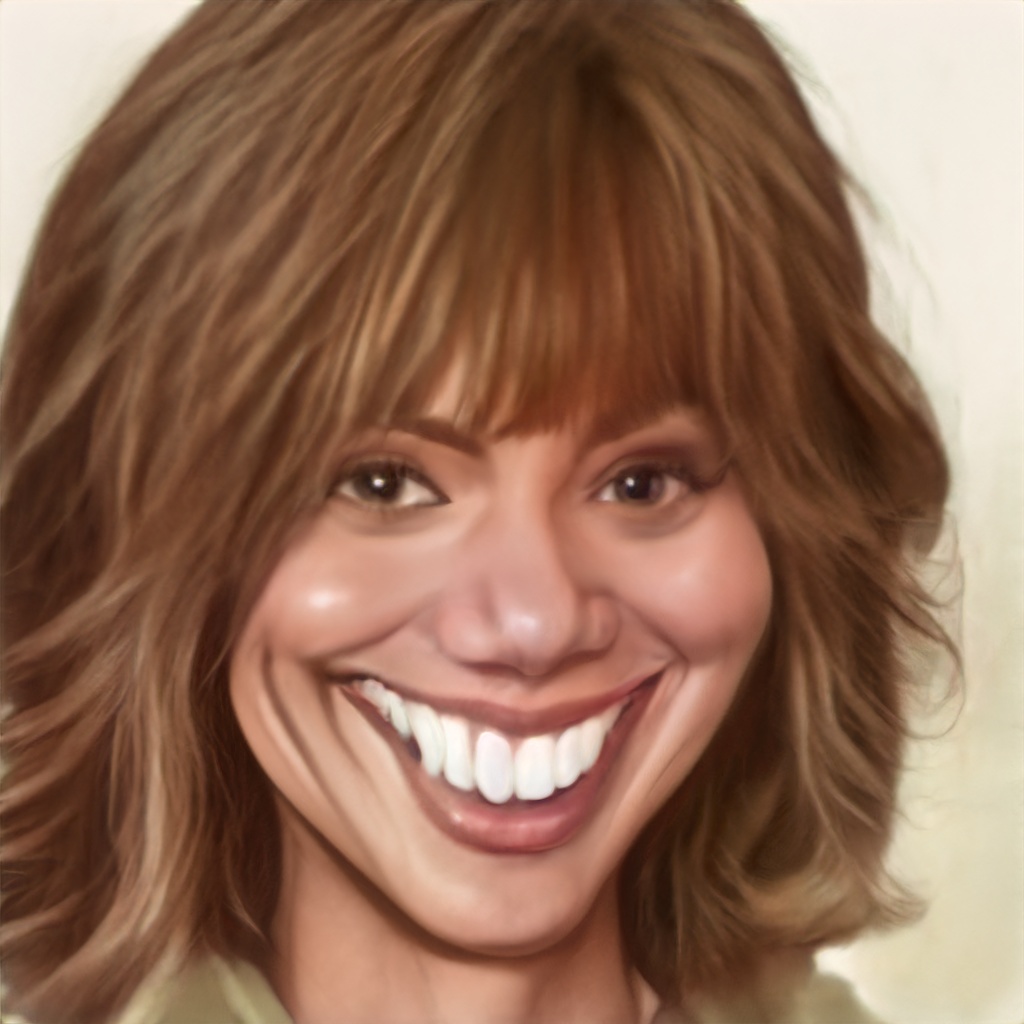
Features
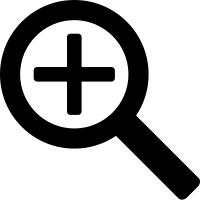
High-Resolution
(1024*1024)
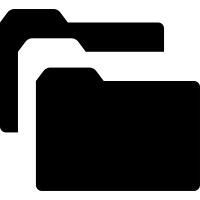
Training Data-Efficient
(100~300 Images)
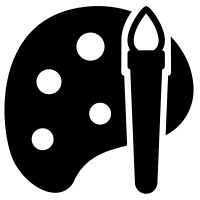
Exemplar-Based
Color Transfer
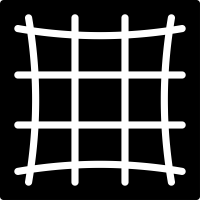
Exemplar-Based
Structure Transfer
The
DualStyleGAN Framework
DualStyleGAN realizes effective modelling and control of dual styles for exemplar-based portrait style transfer. DualStyleGAN retains an intrinsic style path of StyleGAN to control the style of the original domain, while adding an extrinsic style path to model and control the style of the target extended domain, which naturally correspond to the content path and style path in the standard style transfer paradigm. Moreover, the extrinsic style path inherits the hierarchical architecture from StyleGAN to modulate structural styles in coarse-resolution layers and color styles in fine-resolution layers for flexible multi-level style manipulations.
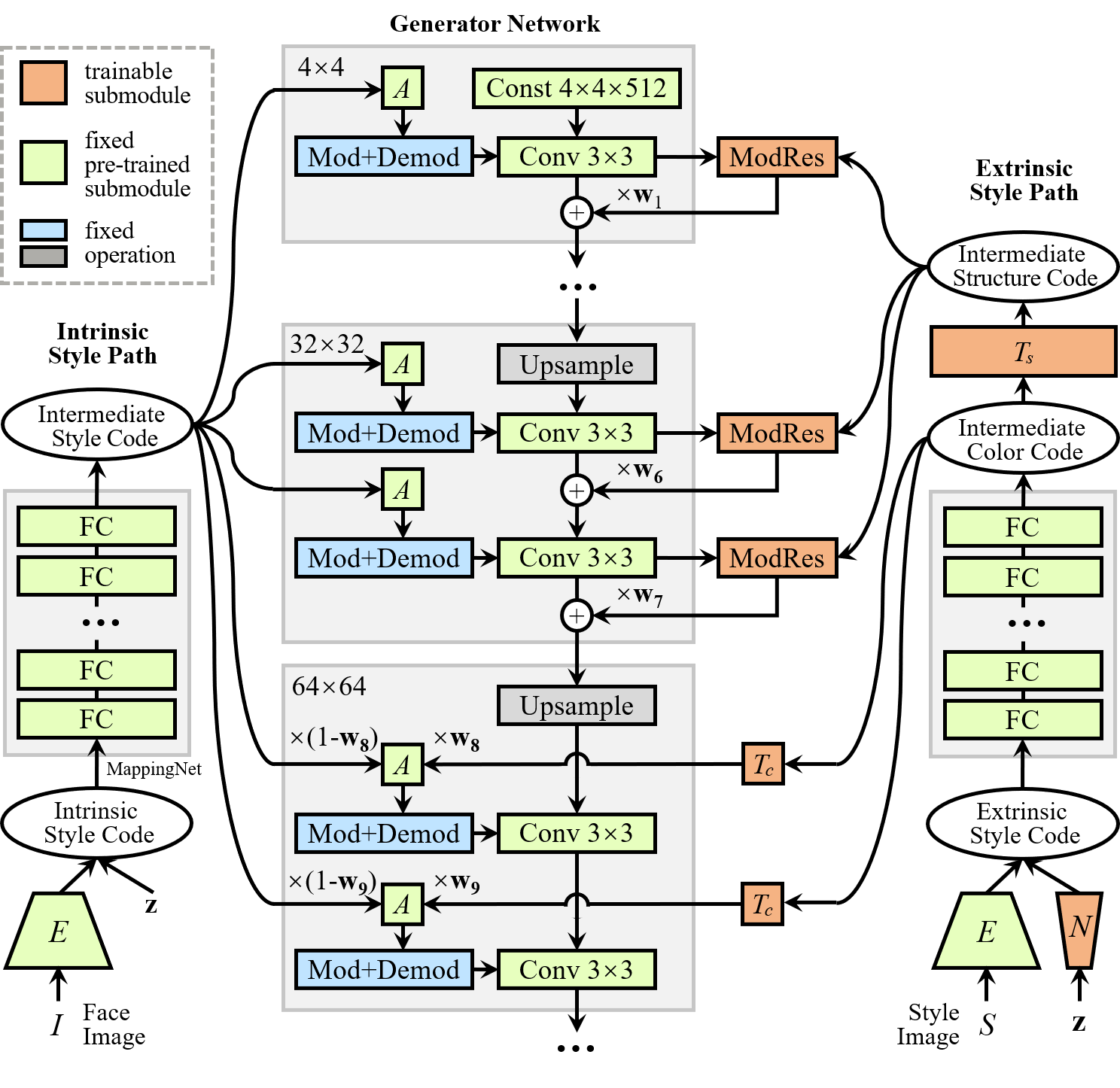
Exemplar-Based
Style Transfer
DualStlyeGAN extends StyleGAN to accept style condition from new domains while preserving its style control in the original domain. This results in an interesting application of high-resolution exemplar-based portrait style transfer with a friendly data requirement. Unlike previous unconditional fine-tuning that translates the StyleGAN generative space as a whole, leading to the loss of diversity of the captured styles, DualStlyeGAN enables users to enlarge the mouth or shrink the face to pastiche their preferred artworks.
Style Types
Artistic Portrait
Generation
We propose a progressive finetuning scheme to extend the generative space of DualStyleGAN from the StyleGAN's photo-realistic human faces to artistic portraits with limited data. By sampling intrinsic and extrinsic style codes, DualStyleGAN generates random artistic portraits of high diversity and high quality.
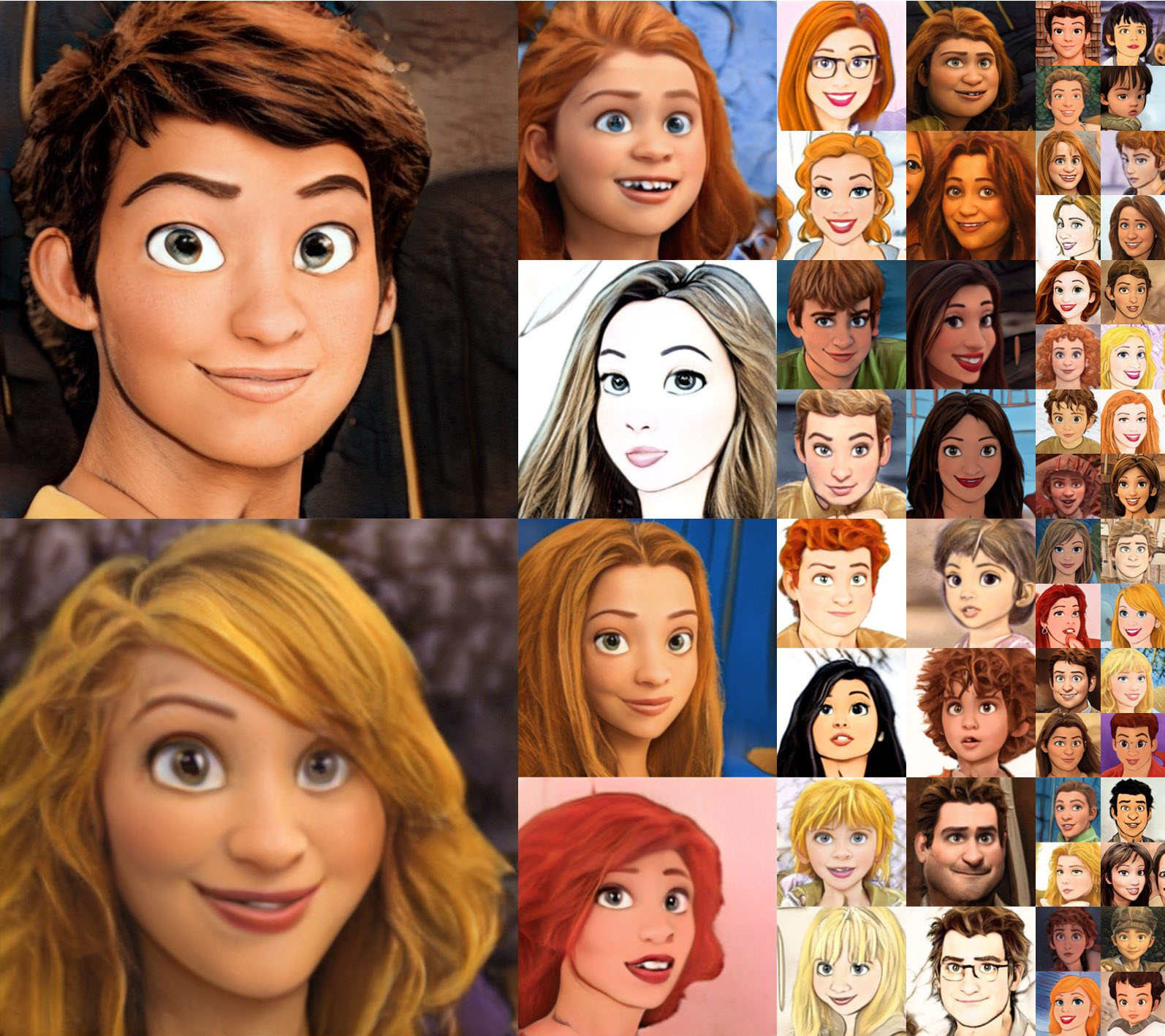
Generated cartoon faces
Illustration of the generative space of DualStyleGAN after being finetuned on 317 cartoon images provided by Toonify.
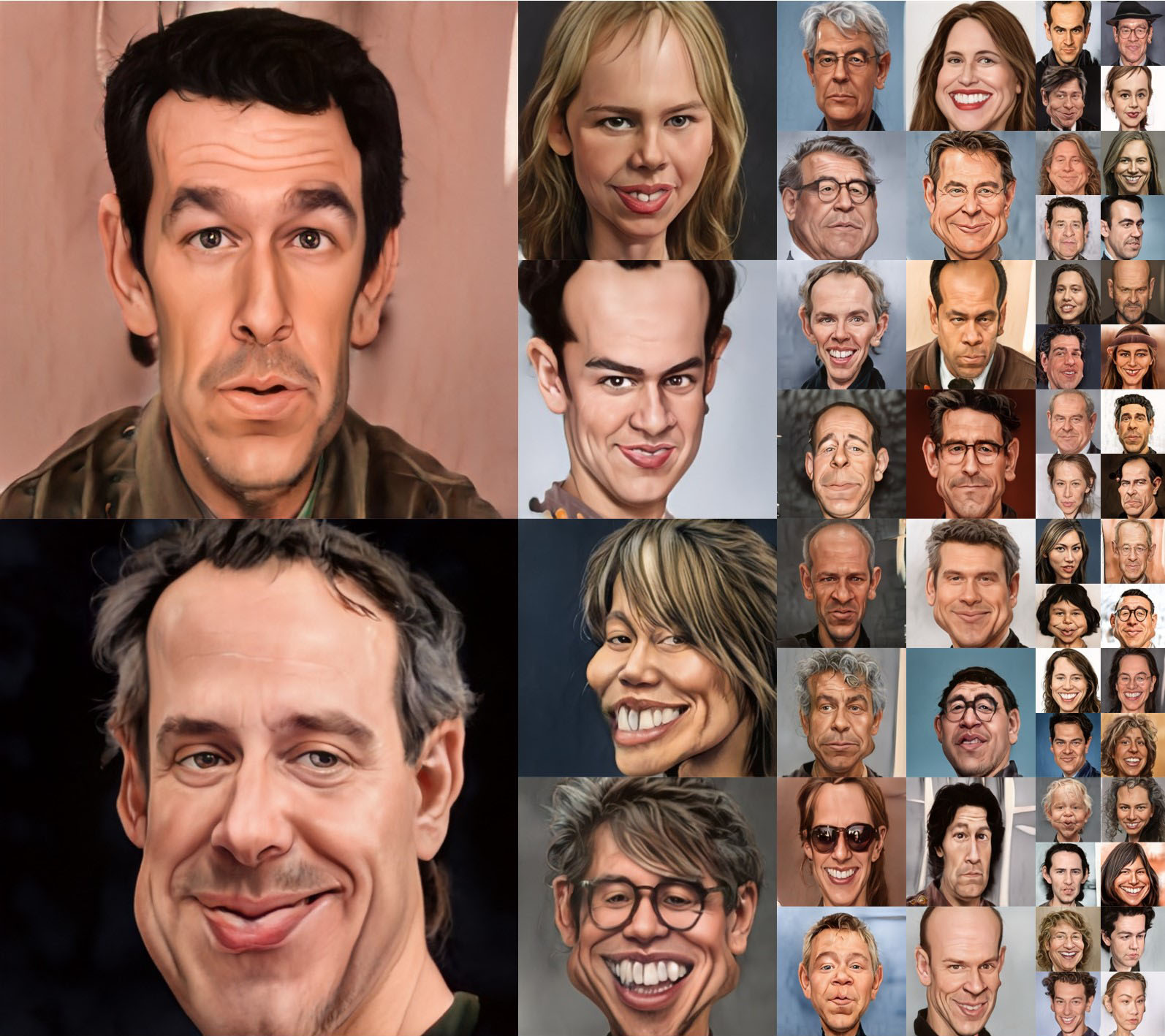
Generated caricature faces
Illustration of the generative space of DualStyleGAN after being finetuned on 199 caricature images from WebCaricature.
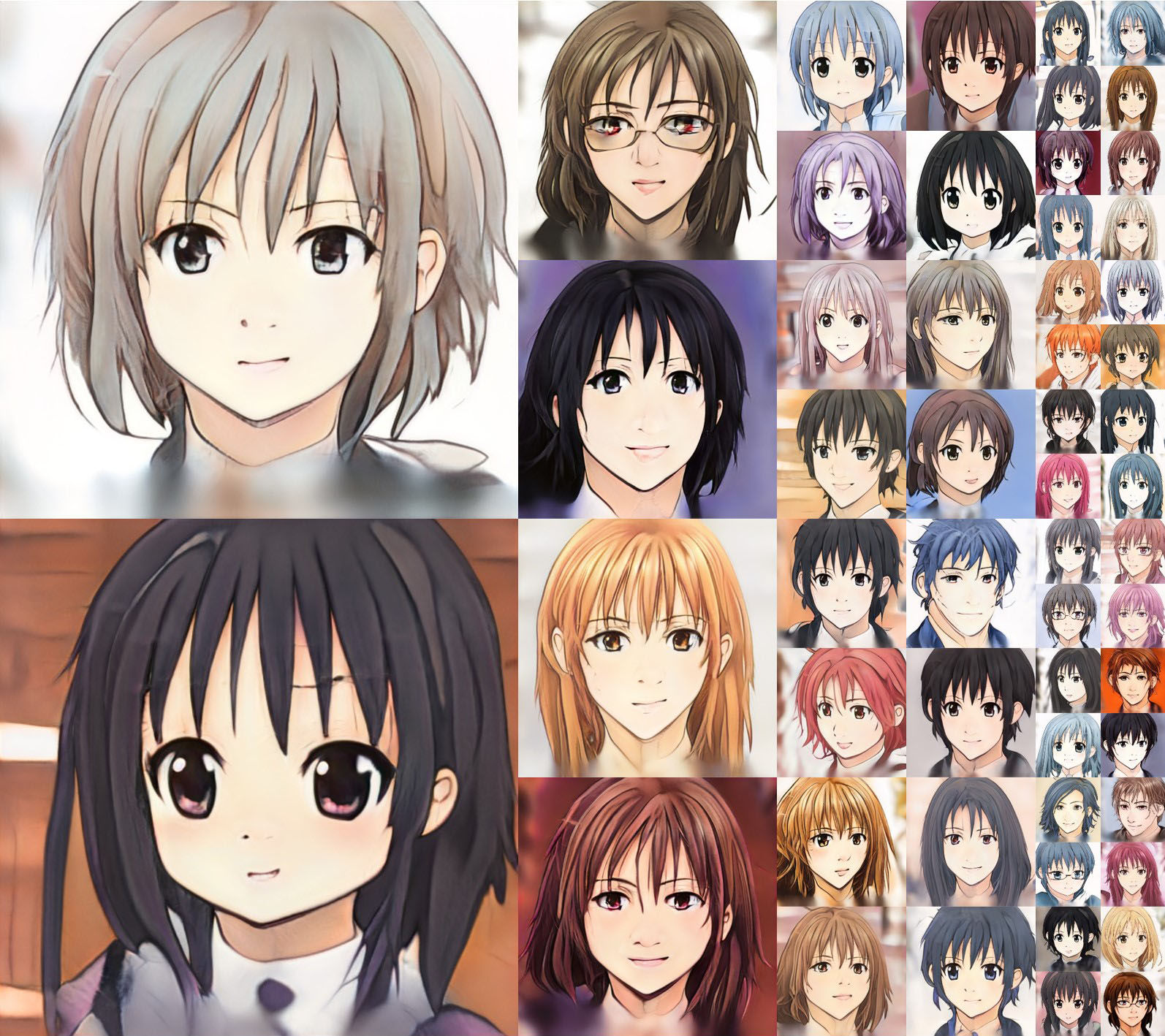
Generated anime faces
Illustration of the generative space of DualStyleGAN after being finetuned on 174 anime images from Danbooru Portraits.
Experimental
Results
Comparison with state-of-the-art methods
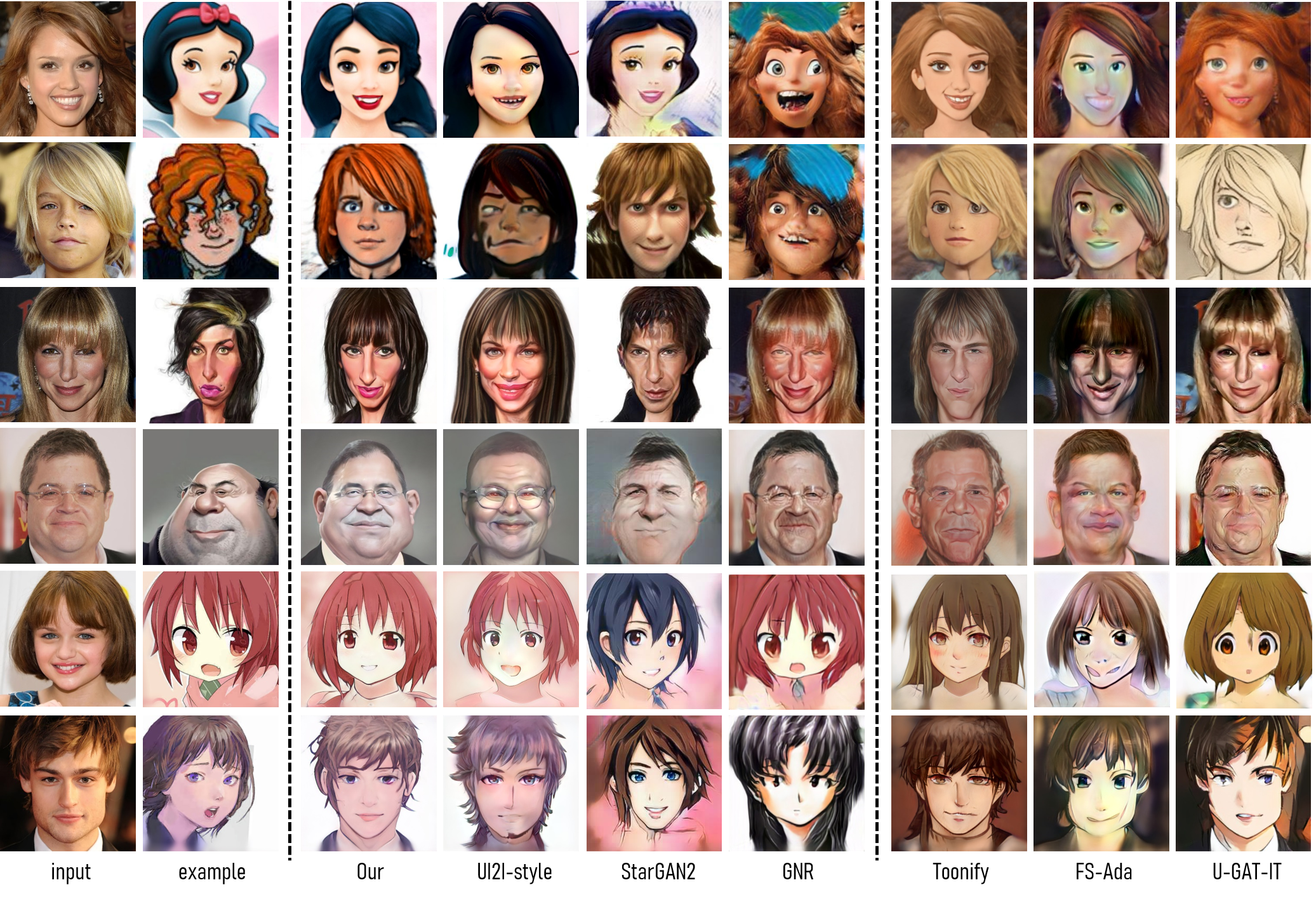
We compare with six state-of-the-art methods: image-to-image-translation-based StarGAN2, GNR, U-GAT-IT, and StyleGAN-based UI2I-style, Toonify, Few-Shot Adaptation (FS-Ada). The image-to-image translation and FS-Ada use 256*256 images. Other methods support 1024*1024. Toonify, FS-Ada and U-GAT-IT learn domain-level rather than image-level styles. Thus their results are not consistent with the style examples. The severe data imbalance problem makes it hard to train valid cycle translations. Thus, StarGAN2 and GNR overfit the style images and ignore the input faces on the anime style. UI2I-style captures good color styles via layer swapping, but the model misalignment makes the structure features hard to blend, leading to failure structural style transfer. By comparison, DualStyleGAN transfers the best style of the exemplar style in both colors and complex structures.
Performance on various styles
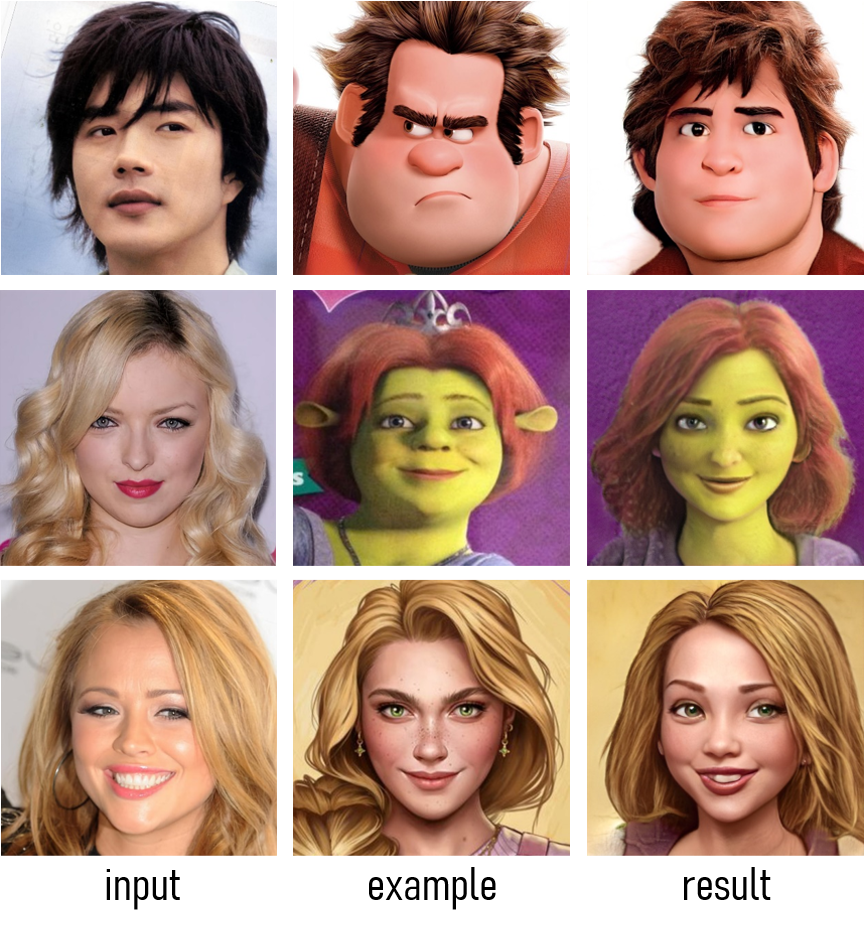
Exemplar-based Cartoon style transfer
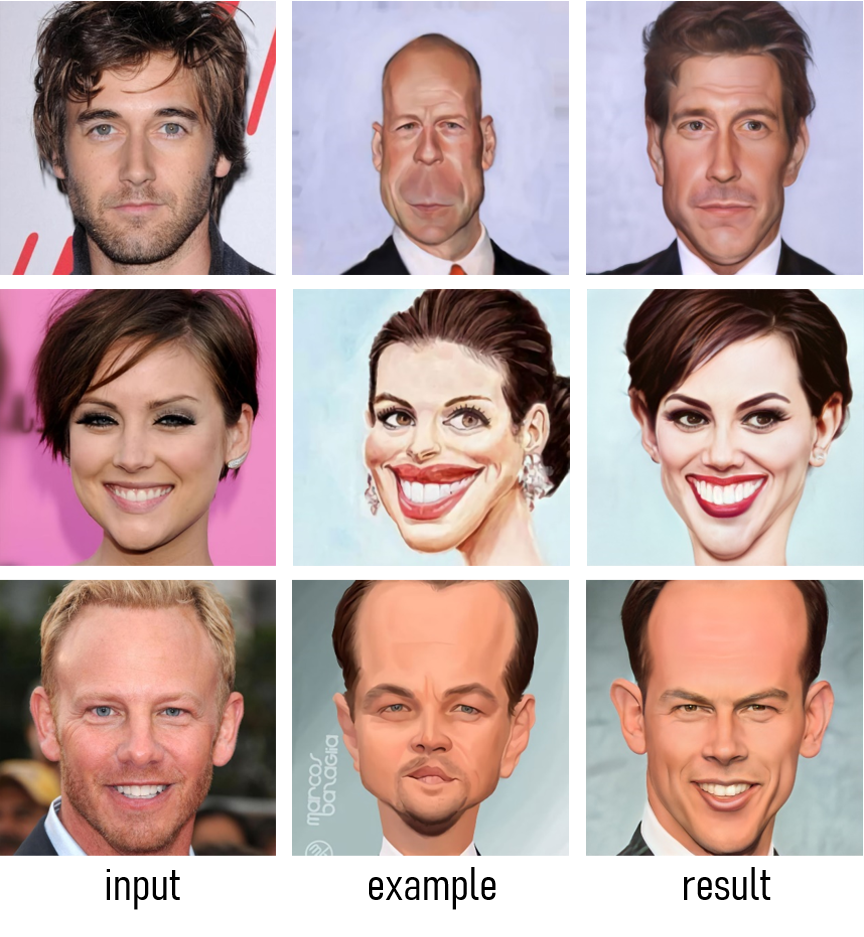
Exemplar-based caricature style trasnfer
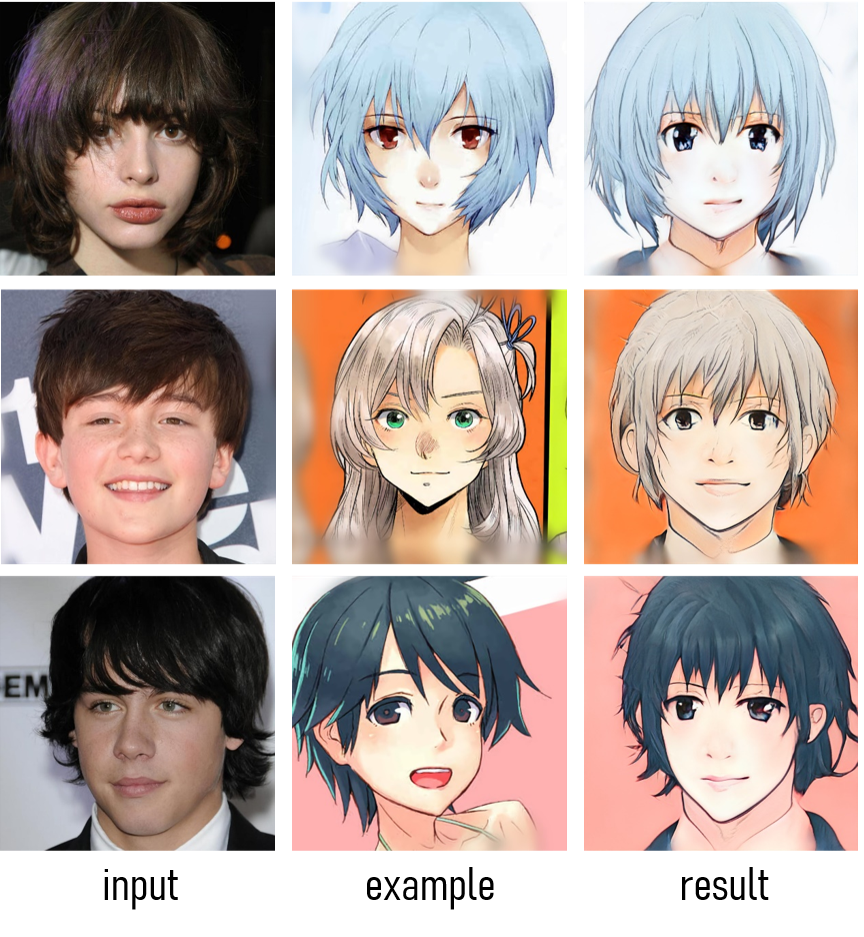
Exemplar-based anime style transfer
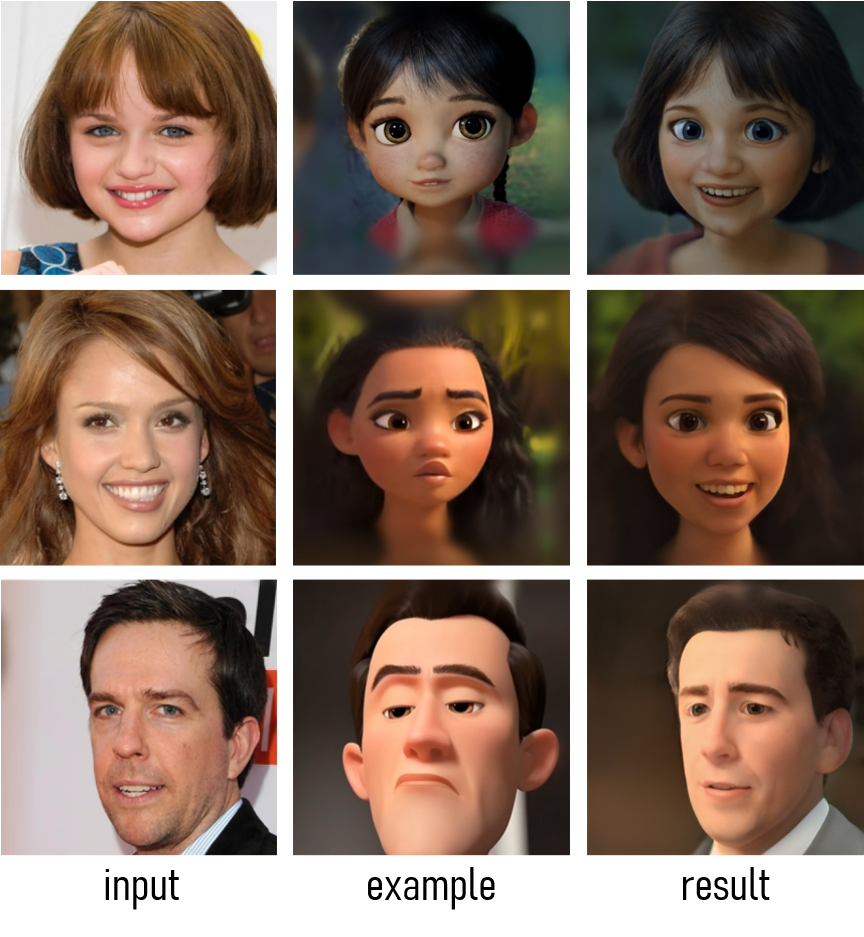
Exemplar-based Pixar style transfer
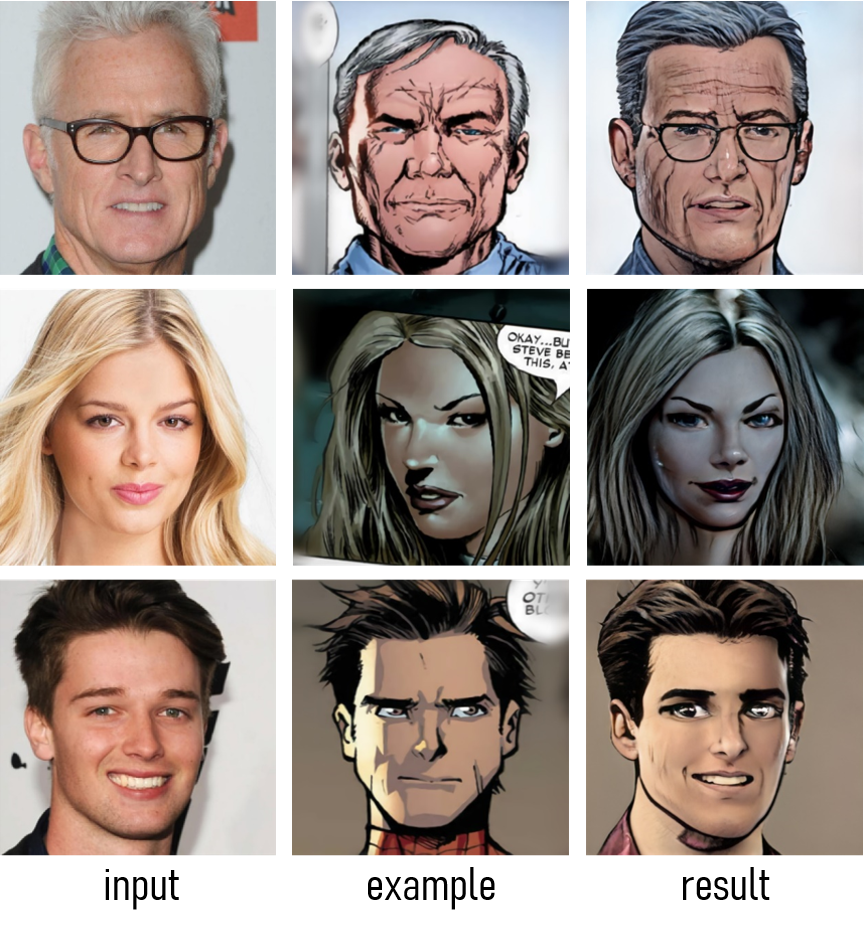
Exemplar-based comic style trasnfer
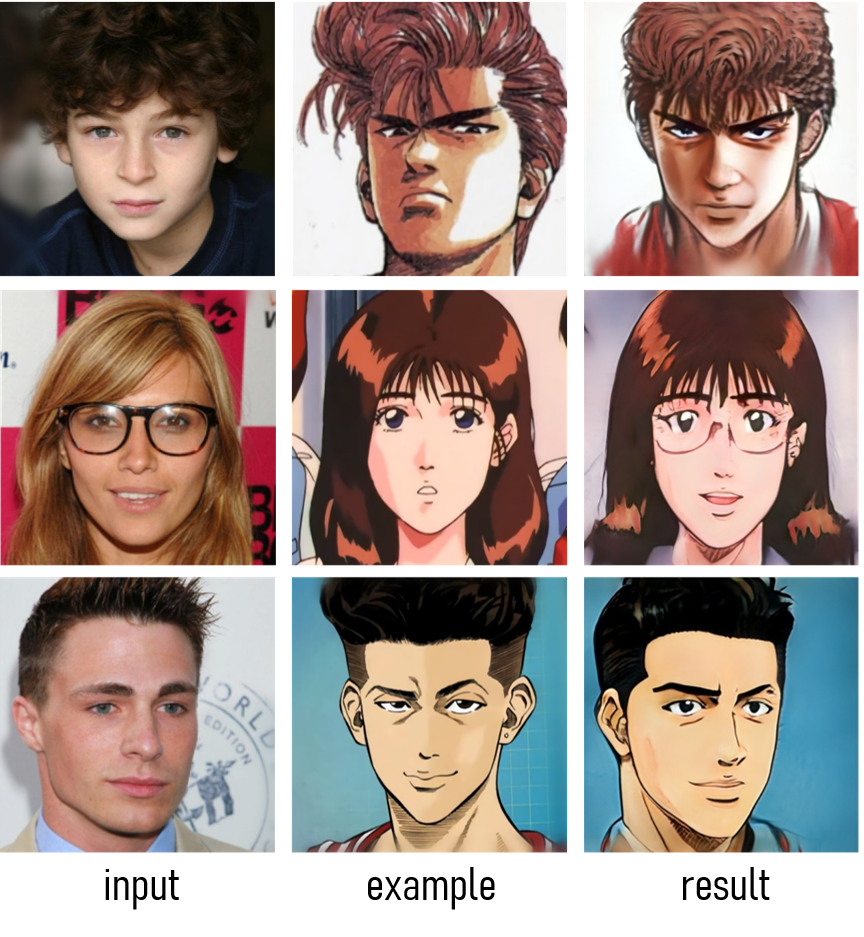
Exemplar-based Slam Dunk style transfer
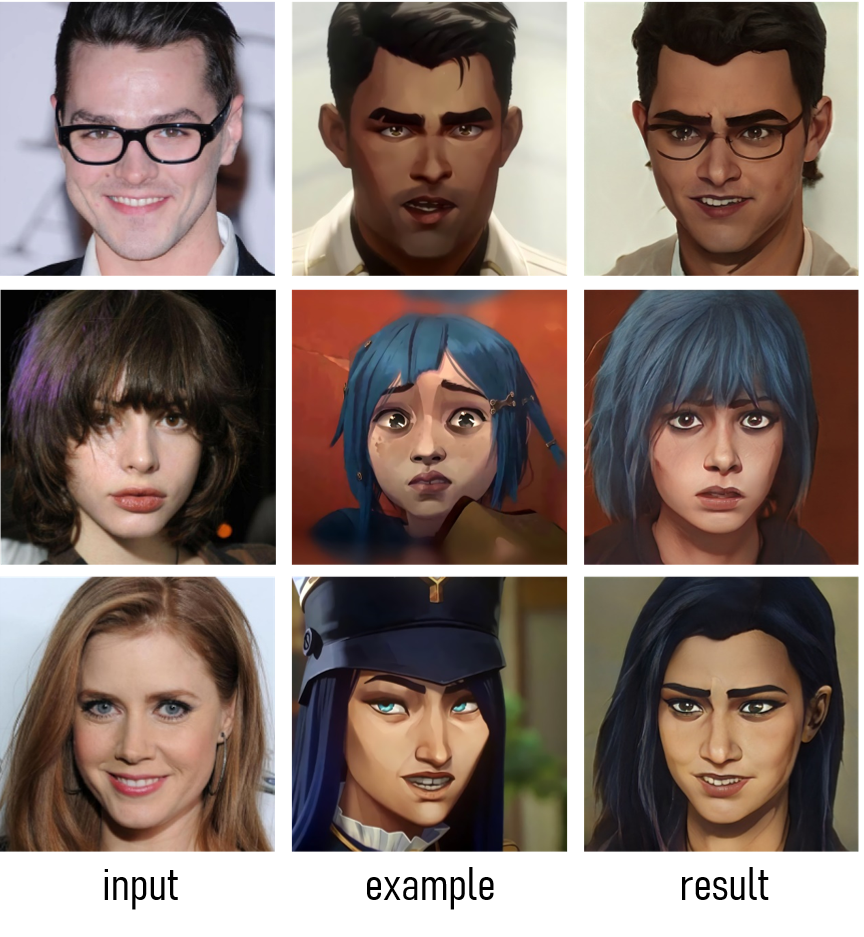
Exemplar-based Arcane style transfer
Paper
Citation
@InProceedings{yang2022Pastiche,
author = {Yang, Shuai and Jiang, Liming and Liu, Ziwei and and Loy, Chen Change},
title = {Pastiche Master: Exemplar-Based High-Resolution Portrait Style Transfer},
booktitle = {Proceedings of the IEEE/CVF Conference on Computer Vision and Pattern Recognition},
year = {2022}
}
Related
Projects
-
Deep Plastic Surgery: Robust and Controllable Image Editing with Human-Drawn Sketches
S. Yang, Z. Wang, J. Liu, Z. Guo
in Proceedings of European Conference on Computer Vision, 2020 (ECCV)
[arXiv] [Project Page] -
Deceive D: Adaptive Pseudo Augmentation for GAN Training with Limited Data
L. Jiang, B. Dai, W. Wu, C. C. Loy
in Proceedings of Neural Information Processing Systems, 2021 (NeurIPS)
[PDF] [arXiv] [YouTube] [Project Page] -
Talk-to-Edit: Fine-Grained Facial Editing via Dialog
Y. Jiang, Z. Huang, X. Pan, C. C. Loy, Z. Liu
in Proceedings of IEEE/CVF International Conference on Computer Vision, 2021 (ICCV)
[PDF] [arXiv] [Project Page]
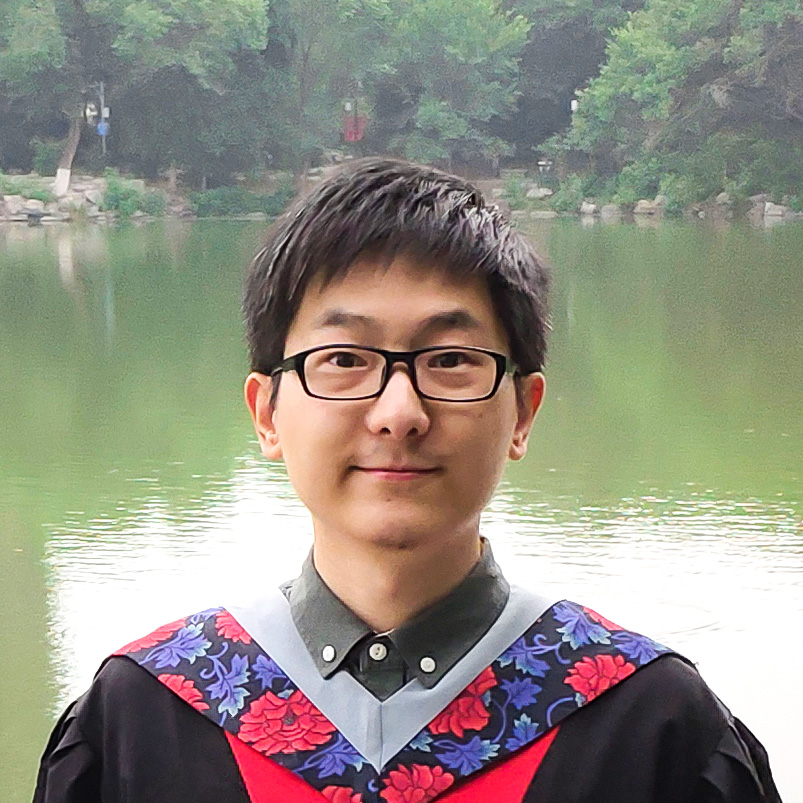