Monocular 3D Object Reconstruction
with GAN Inversion
ECCV 2022
Paper
Abstract
Recovering a textured 3D mesh from a monocular image is highly challenging, particularly for in-the-wild objects that lack 3D ground truths. In this work, we present MeshInversion, a novel framework to improve the reconstruction by exploiting the generative prior of a 3D GAN pre-trained for 3D textured mesh synthesis. Reconstruction is achieved by searching for a latent space in the 3D GAN that best resembles the target mesh in accordance with the single view observation. Since the pre-trained GAN encapsulates rich 3D semantics in terms of mesh geometry and texture, searching within the GAN manifold thus naturally regularizes the realness and fidelity of the reconstruction. Importantly, such regularization is directly applied in the 3D space, providing crucial guidance of mesh parts that are unobserved in the 2D space. Experiments on standard benchmarks show that our framework obtains faithful 3D reconstructions with consistent geometry and texture across both observed and unobserved parts. Moreover, it generalizes well to meshes that are less commonly seen, such as the extended articulation of deformable objects.
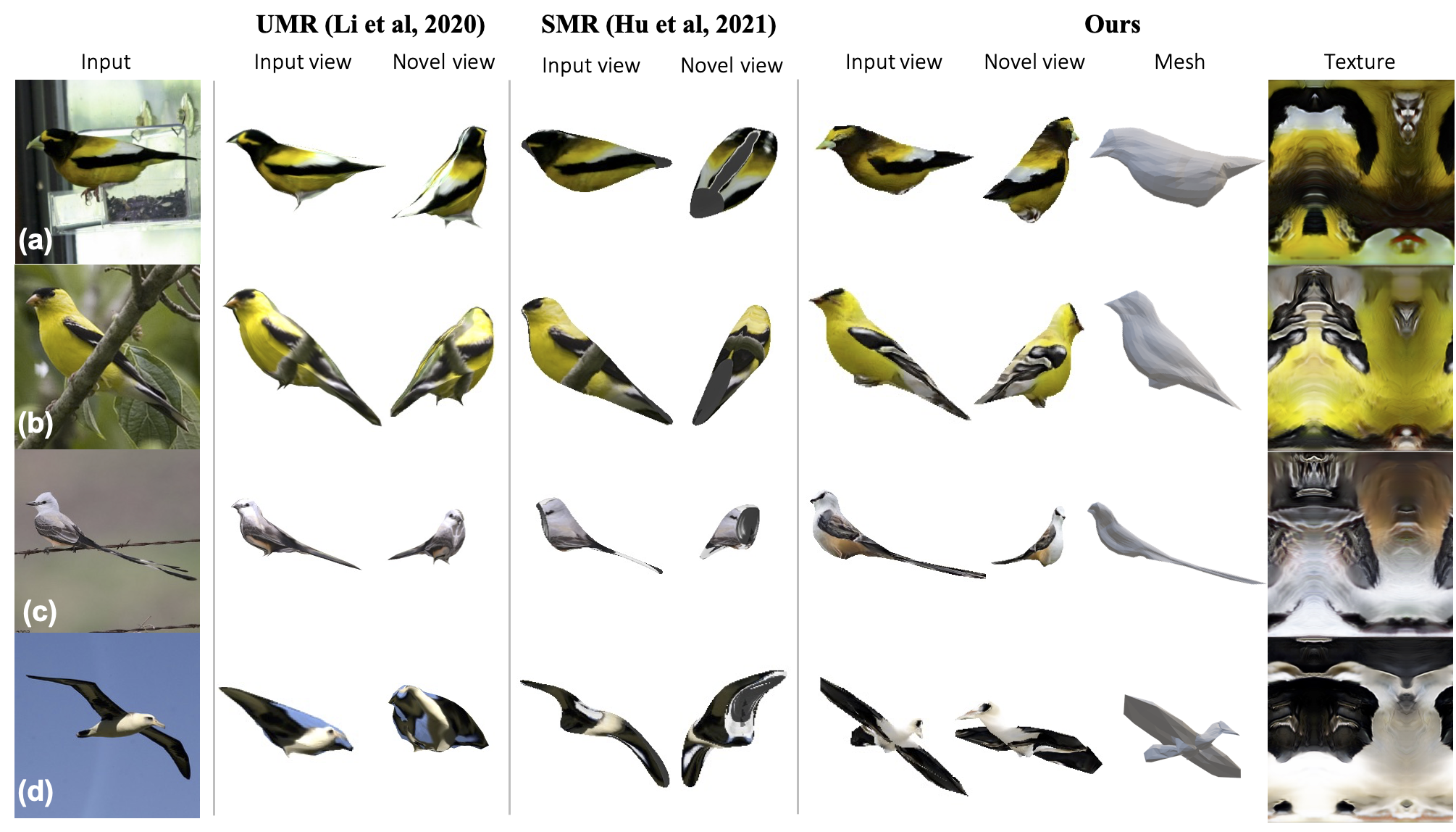
We propose an alternative approach to monocular 3D reconstruction by exploiting generative prior encapsulated in a pre-trained GAN. Our method has three major advantages: 1) It reconstructs highly faithful and realistic 3D objects, even when observed from novel views; 2) The reconstruction is robust against occlusion in (b); 3) The method generalizes reasonably well to less common shapes, such as birds with (c) extended tails or (d) open wings.
MeshInversion
Framework
We reconstruct a 3D object from its monocular observation by incorporating a pre-trained textured 3D GAN G. We search for the latent code z and fine-tune the imperfect camera that minimizes 2D reconstruction losses via gradient descent. To address the intrinsic challenges associated with 3D-to-2D degradation, we propose two Chamfer-based losses: 1) Chamfer Texture Loss relaxes the pixel-wise correspondences between two RGB images or feature maps, and factorizes the pairwise distance into spatial and textural distance terms. We illustrate the distance maps between one anchor point from the rendered image to the input image, where brighter regions correspond to smaller distances. 2) Chamfer Mask Loss intercepts the discretization process and computes the Chamfer distance between the projected vertices and the foreground pixels projected to the same continuous space.
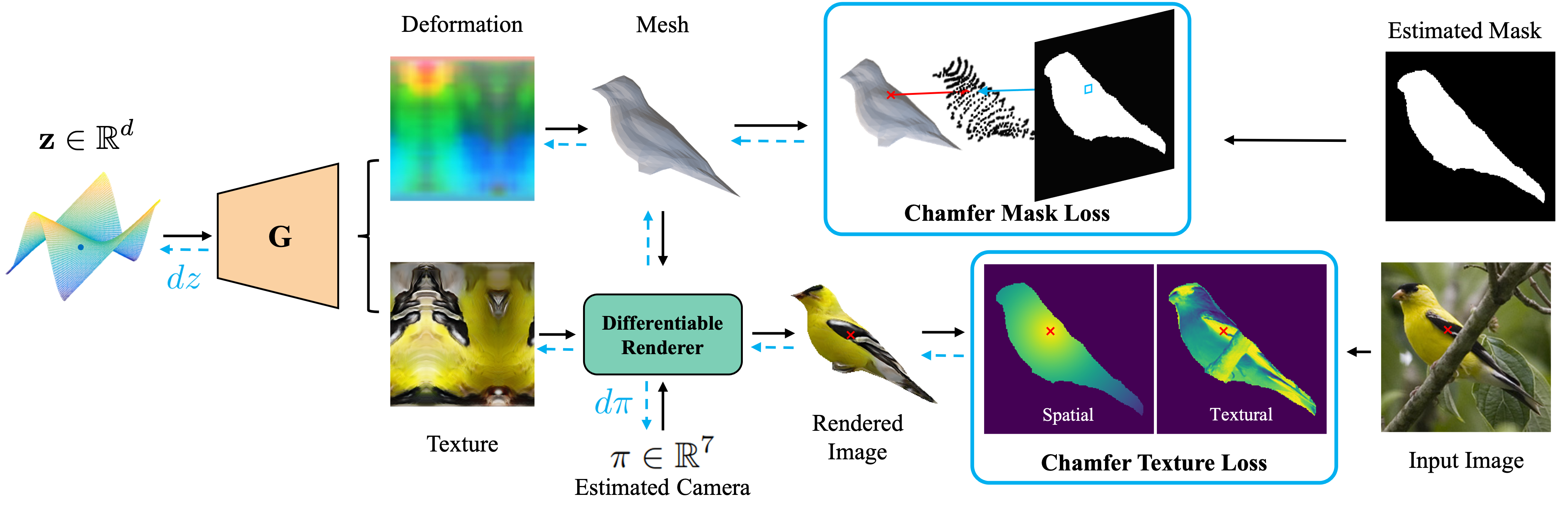
Qualitative Results
CUB-200-2011 Dataset
Our method achieves highly faithful and realistic 3D reconstruction. In particular, it exhibits superior generalization under various challenging scenarios, including with occlusion (b, c) and extended articulation (d, e).
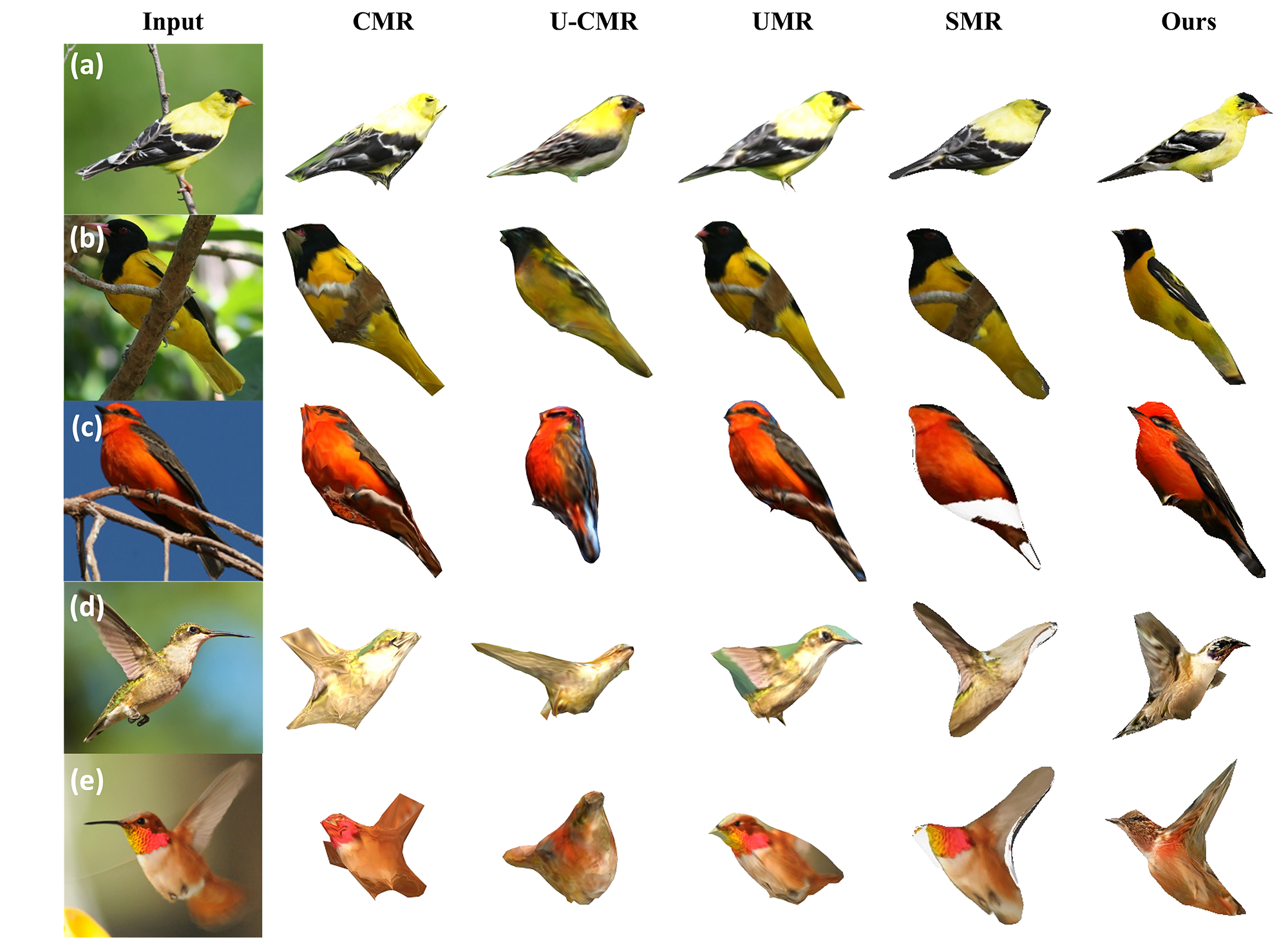
Novel-view
Rendering
Our method gives realistic and faithful 3D reconstruction in terms of both 3D shape and appearance. It generalizes fairly well to invisible regions and challenging articulations.
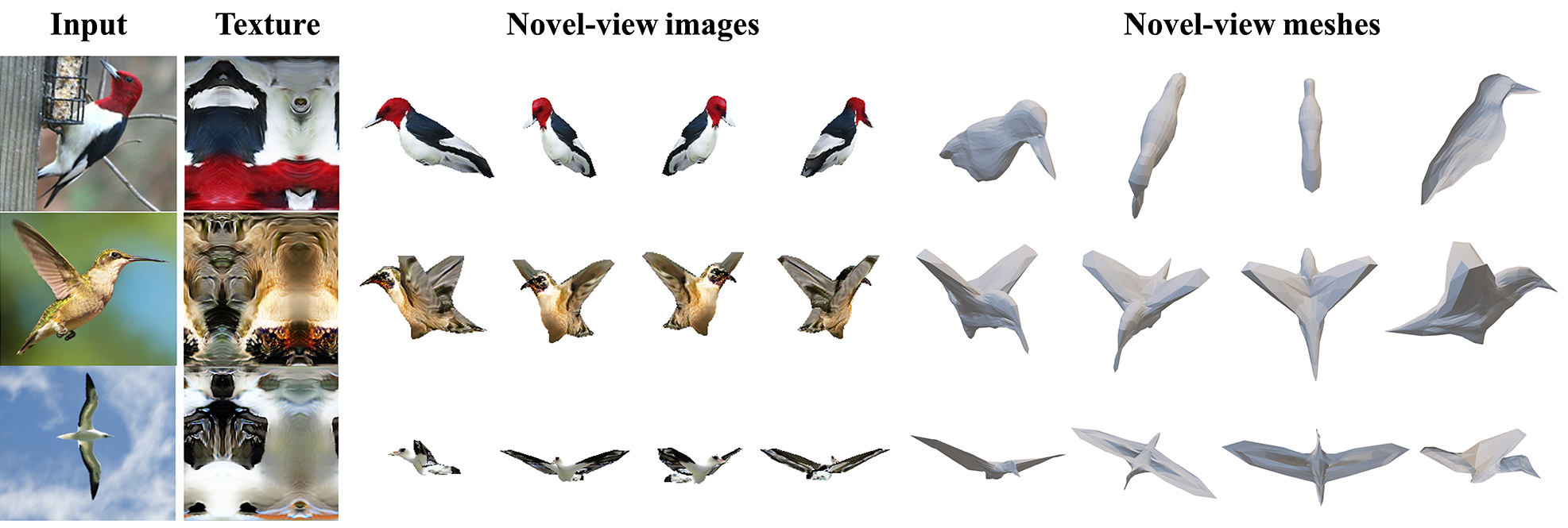
Texture
Transfer
MeshInversion enables faithful and realistic texture transfer between bird instances even with highly articulated shapes.
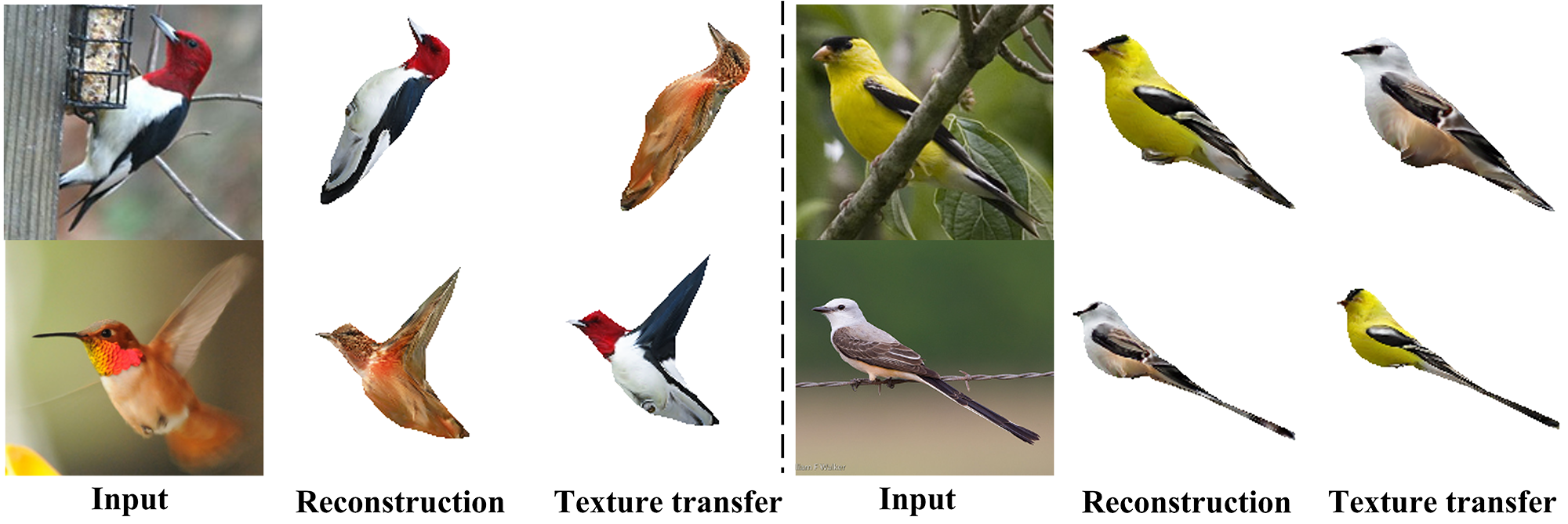
Paper
Citation
@inproceedings{zhang2022monocular,
title = {Monocular 3D Object Reconstruction with GAN Inversion},
author = {Zhang, Junzhe and Ren, Daxuan and Cai, Zhongang and Yeo, Chai Kiat and Dai, Bo and Loy, Chen Change},
booktitle = {European Conference on Computer Vision},
year = {2022}}
Related
Projects
-
Unsupervised 3D Shape Completion through GAN Inversion
J. Zhang, X. Chen, Z. Cai, L. Pan, H. Zhao, S. Yi, C. K. Yeo, B. Dai, C. C. Loy
in Proceedings of IEEE Conference on Computer Vision and Pattern Recognition, 2021 (CVPR)
[Paper] [Project Page] -
Do 2D GANs Know 3D Shape? Unsupervised 3D Shape Reconstruction from 2D Image GANs
X. Pan, B. Dai, Z. Liu, C. C. Loy, P. Luo
in International Conference on Learning Representations, 2021 (ICLR)
[Paper] [Project Page] -
Exploiting Deep Generative Prior for Versatile Image Restoration and Manipulation
X. Pan, X. Zhan, B. Dai, D. Lin, C. C. Loy, P. Luo
in IEEE Transactions on Pattern Analysis and Machine Intelligence, 2021 (TPAMI)
[Paper] [Project Page]
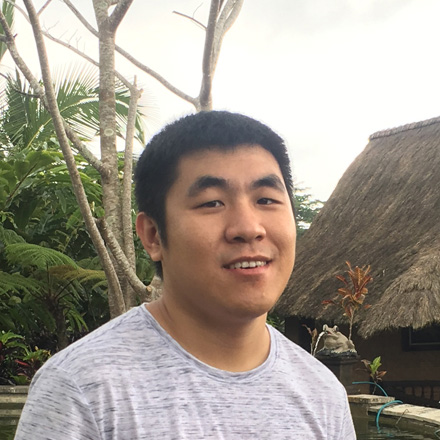