Talk-to-Edit:
Fine-Grained Facial Editing via Dialog
ICCV 2021
Paper
Abstract
Facial editing is an important task in vision and graphics with numerous applications. However, existing works are incapable to deliver a continuous and fine-grained editing mode (e.g., editing a slightly smiling face to a big laughing one) with natural interactions with users. In this work, we propose Talk-to-Edit, an interactive facial editing framework that performs fine-grained attribute manipulation through dialog between the user and the system. Our key insight is to model a continual "semantic field" in the GAN latent space. 1) Unlike previous works that regard the editing as traversing straight lines in the latent space, here the fine-grained editing is formulated as finding a curving trajectory that respects fine-grained attribute landscape on the semantic field. 2) The curvature at each step is location-specific and determined by the input image as well as the users' language requests. 3) To engage the users in a meaningful dialog, our system generates language feedback by considering both the user request and the current state of the semantic field.
We also contribute CelebA-Dialog, a visual-language facial editing dataset to facilitate large-scale study. Specifically, each image has manually annotated fine-grained attribute annotations as well as template-based textual descriptions in natural language. Extensive quantitative and qualitative experiments demonstrate the superiority of our framework in terms of 1) the smoothness of fine-grained editing, 2) the identity/attribute preservation, and 3) the visual photorealism and dialog fluency. Notably, user study validates that our overall system is consistently favored by around 80% of the participants.
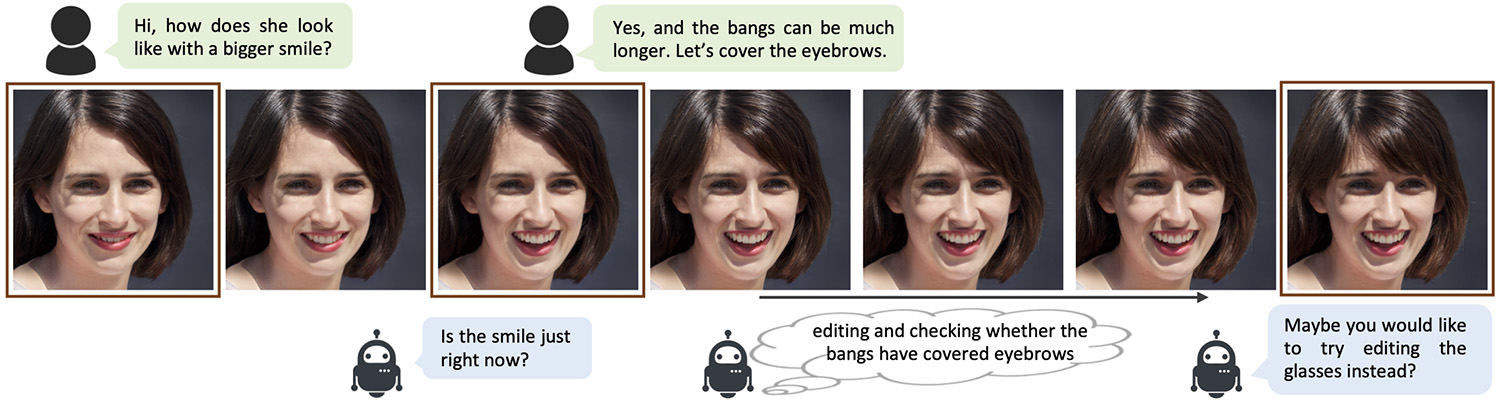
We propose Talk-to-Edit, an interactive facial editing framework that performs fine-grained facial editing through dialog between the user and the system.
The Pipeline
Talk-to-Edit
The pipeline consists of three components:
- Language Encoder: understands user request.
- Semantic Field: performs fine-grained editing.
- Talk Module: provides meaningful natural language feedback.
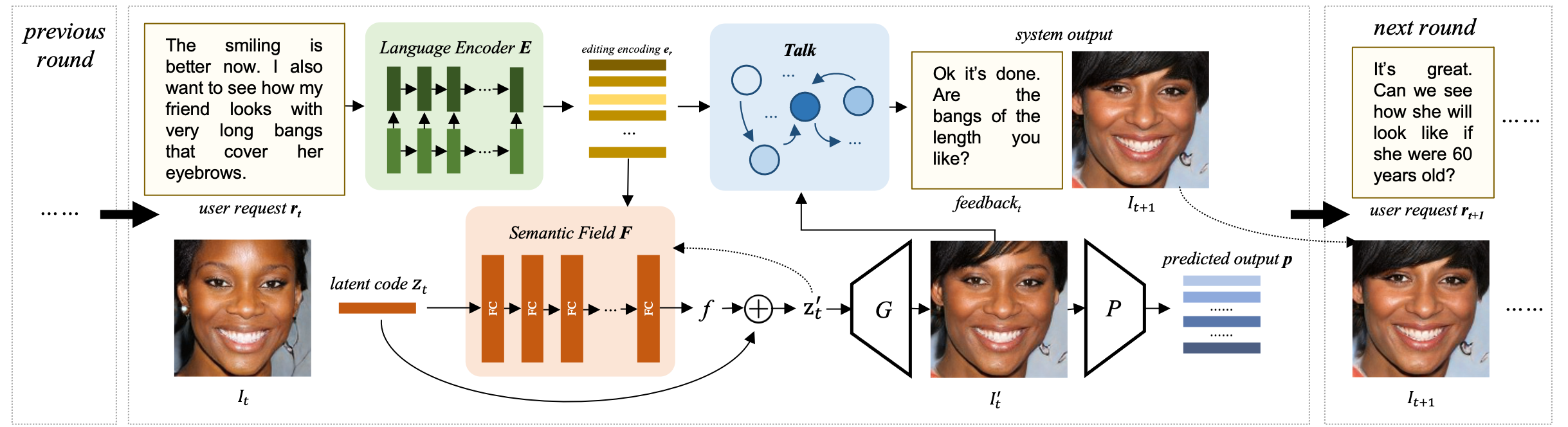
The
Semantic Field
In the StyleGAN latent space, the attribute score is a scalar field. The gradient of attribute score field with respect to the latent code is a vector field, which we term as “semantic field”. We learn the semantic field, and move the latent code along the learned field lines to achieve facial editing.
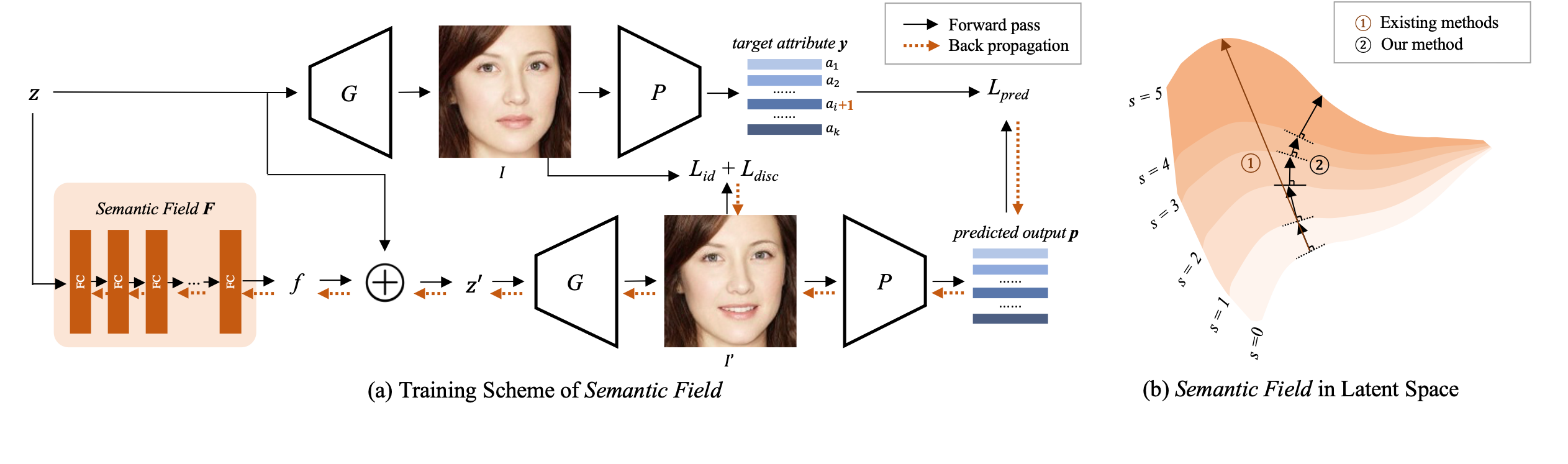
The Dataset
CelebA-Dialog
We contribute a large-scale visual-language face dataset named CelebA-Dialog:
- Facial images are annotated with rich fine-grained labels, which classify one attribute into multiple degrees according to its semantic meaning.
- Accompanied with each image, there are captions describing the attributes and a user request sample.
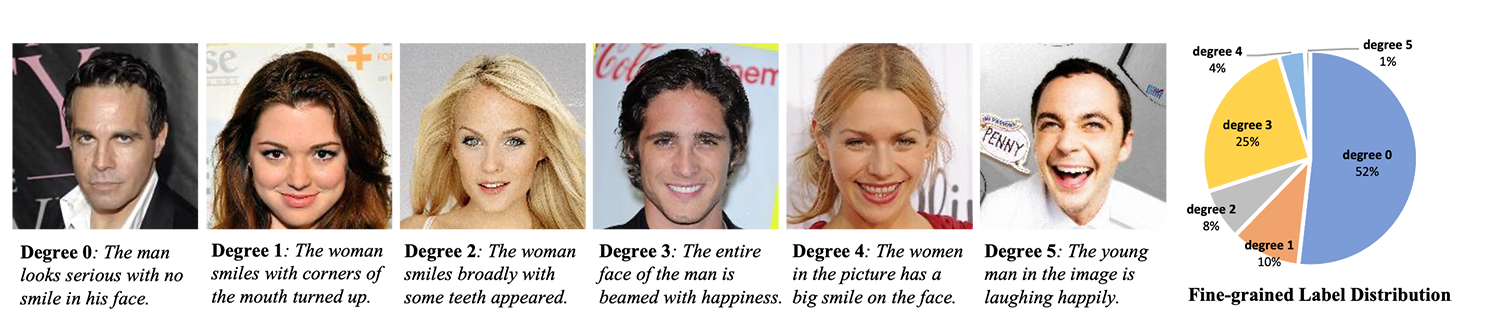
Illustration of CelebA-Dialog dataset. We show example images and annotations for the smiling attribute. Below the images are the attribute degrees and the corresponding textual descriptions. We also show the fine-grained label distribution of the smiling attribute.
Qualitative
Results
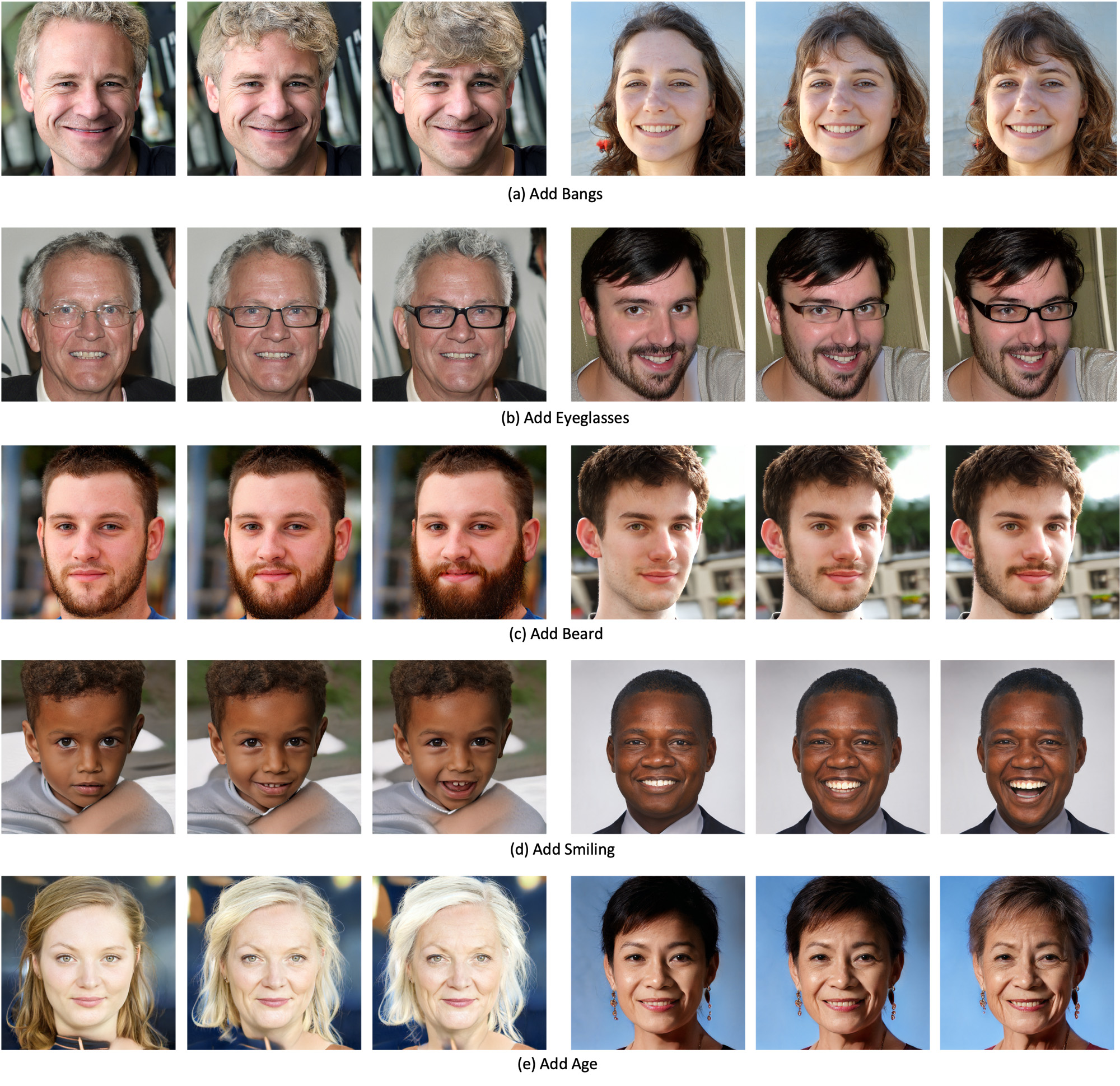
Qualitative results on the manipulation of five attributes respectively: Bangs, Eyeglasses, Beard, Smiling, Young.
Paper
Citation
@InProceedings{jiang2021talkedit,
author = {Jiang, Yuming and Huang, Ziqi and Pan, Xingang and Loy, Chen Change and Liu, Ziwei},
title = {Talk-to-Edit: Fine-Grained Facial Editing via Dialog},
booktitle = {Proceedings of the IEEE/CVF International Conference on Computer Vision},
year = {2021}
}
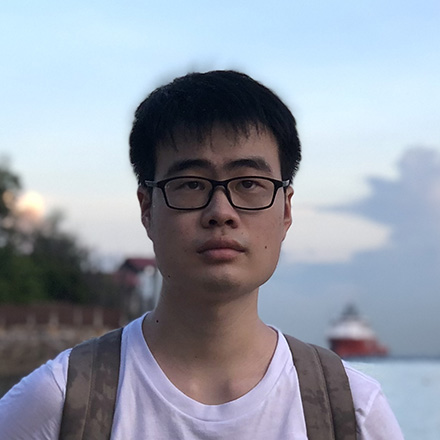
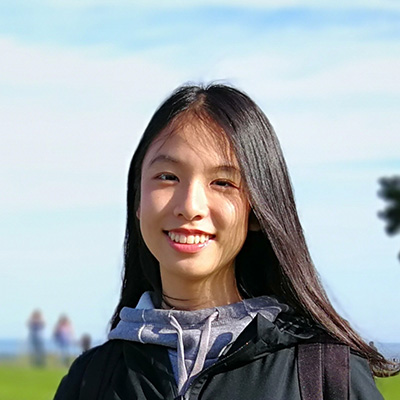